Sentiment Analysis on YouTube Comments With Comments Analytics
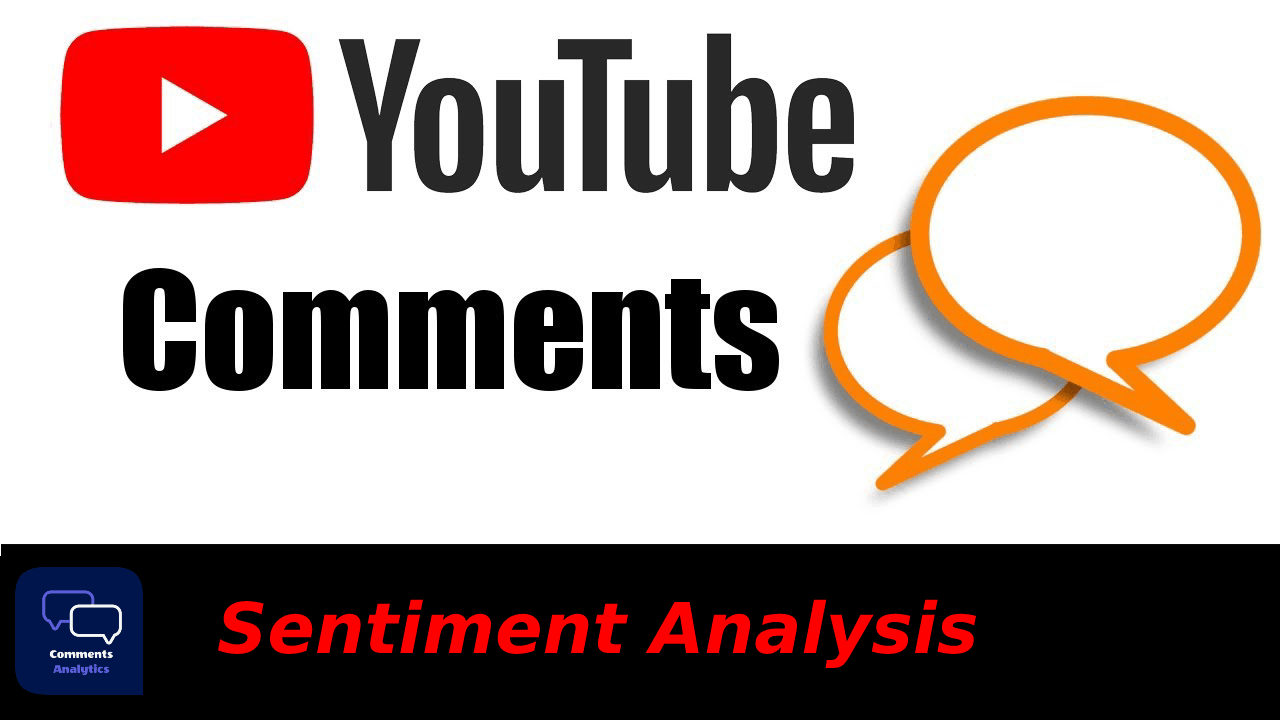
Sentiment Analysis is the process of using natural language processing, text analysis, and computational linguistics to identify and extract subjective information from source materials. In the context of business, sentiment analysis is a powerful tool for gaining insights into customer opinions, needs , and behaviors. By analyzing customer feedback, businesses can identify patterns and trends that may not be immediately apparent through more traditional forms of data analysis, and use this information to make more informed decisions and improve their products, services, and overall customer experience.
Business-Driven Sentiment Analysis:
One of the primary applications of sentiment analysis in business is in customer relationship management. By analyzing customer feedback, businesses can gain a deeper understanding of how their customers feel about their products, services, and overall brand. This can help companies identify issues and opportunities, and take steps to address them. For example, if a large number of customers are expressing frustration with a particular product feature, sentiment analysis can help a company identify this issue and take steps to address it. Additionally, by continuously monitoring customer feedback, businesses can quickly identify emerging issues and opportunities and respond in a timely and appropriate manner, which can help to build stronger relationships with customers and ultimately improve customer loyalty and retention.
Another application of sentiment analysis in business is in marketing and advertising. By analyzing the sentiment expressed in response to marketing materials and campaigns, businesses can identify what is and is not resonating with their target audience, and adjust their approach accordingly. Sentiment analysis can also be used to monitor the effectiveness of marketing campaigns by tracking the sentiment of customer feedback over time. This can help companies to identify areas where their campaigns are succeeding and where they need to be improved.
Sentiment analysis can also be used in product development. By analyzing customer feedback, businesses can identify which features of their products are most popular and which are causing the most frustration. This can help companies to prioritize development efforts and make more informed decisions about which features to develop next. Additionally, Sentiment analysis can be used in industries like e-commerce, social media, and customer service to track the general perception of their brand, monitor the effectiveness of their customer support, and track the reputation of the brand.
Youtube comments sentiment analysis applications with "Comments Analysis" on YouTube service:
YouTube video comments sentiment analysis is an important tool for businesses looking to gain insights into customer opinions and feedback. By analyzing the comments left on their videos, businesses can gain a deeper understanding of how their customers feel about their products, services, and overall brand. This information can be used to identify issues and opportunities, and take steps to address them. For example, if a large number of customers are expressing frustration with a particular product feature, sentiment analysis can help a company identify this issue and take steps to address it. Additionally, by continuously monitoring customer feedback, businesses can quickly identify emerging issues and opportunities and respond in a timely and appropriate manner, which can help to build stronger relationships with customers and ultimately improve customer loyalty and retention.
YouTube video comments sentiment analysis is also valuable for businesses in the context of marketing and advertising. By analyzing the sentiment expressed in response to marketing materials and campaigns, businesses can identify what is and is not resonating with their target audience, and adjust their approach accordingly. Sentiment analysis can also be used to monitor the effectiveness of marketing campaigns by tracking the sentiment of customer feedback over time. This can help companies to identify areas where their campaigns are succeeding and where they need to be improved. By understanding the sentiment of comments on their videos, businesses can optimize their marketing efforts, find the best ways to connect with their audiences and gain deeper insights into their target market.
Chrome Extension tool: The best tool to export Youtube video comments:
Using a chrome extension of Comments Analytics is an efficient way to export comments from YouTube videos easily. The chrome extension can be installed as an add-on to the Chrome browser, and once installed, it allows users to easily select and extract comments from a YouTube video with a simple click of a button. The tool can then export the comments in a format that is easy to analyze and understand, such as a spreadsheet. Additionally, the chrome extension can also allow for the filtering, sorting and tagging of the comments for more specific analysis, making it easy for businesses to extract and analyze customer feedback from various sources, such as YouTube videos, to gain insights.
Usage Tip
Once the comments have been exported, they can be easily imported into the Comments Analytics dashboard. The dashboard is a user-friendly platform that allows for easy analysis of the comments and provides various analysis options, such as Keyword extraction and sentiment analysis. The dashboard also allows users to create a "Bulk Request" from these exported comments. This feature can help businesses to identify patterns and trends that may not be immediately apparent through more traditional forms of data analysis.The combination of the chrome extension and the Comments Analytics dashboard provides businesses with a powerful tool for extracting, analyzing, and gaining insights from YouTube comments. The chrome extension makes it easy to export comments from YouTube videos, and the dashboard provides a platform for easy analysis of the comments and visualization of the data. By using this tool, businesses can stay attuned to the needs and opinions of their customers, and make informed decisions about how to improve their products and services. Additionally, this tool can also help businesses to track their brand reputation by monitoring the comments on their videos and general perception of their brand, which can have a significant impact on their overall business performance.
Social media comments sentiment analysis benefits by CommentsAnalytics:
sentiment analysis in social media comments can be a valuable tool for businesses looking to gain insights into customer opinions and feedback. Some important benefits of using this service are listed as follows:
- Identifying customer feedback and sentiment towards products, services and overall brand
- Identifying customer issues and opportunities and addressing them in timely manner
- Improving customer loyalty and retention
- Optimizing marketing efforts and connecting with target audiences
- Monitoring brand reputation and tracking sentiment over time
- Prioritizing product development efforts based on customer feedback
- Filtering, sorting, and tagging comments for specific analysis
- Easy to use platform with visualization options such as charts and graphs
- Extracting and analyzing comments from multiple social media platforms
- Customized reporting and data export options
Simple steps to obtain sentiment info from Youtube comments:
Famouse sentiment analysis methods in NLP:
Sentiment analysis has become an increasingly popular tool for businesses looking to gain insight into customer opinions and feedback, as well as for researchers seeking to understand the attitudes and emotions of people in online communities. There are three popular sentiment analysis methods in machine learning: lexicon-based methods, machine learning-based methods, and deep learning-based methods.
lexicon-based sentiment analysis
The first of these methods is lexicon-based sentiment analysis, which involves using a pre-defined lexicon of words and their associated sentiment scores to classify the sentiment of a given text. This method is simple and fast, and it can provide accurate results in many cases. However, it can also be limited by the quality and coverage of the lexicon, and it may not be able to capture the nuances and subtleties of sentiment.
ML-based sentiment analysis
The second method is machine learning-based sentiment analysis, which involves using algorithms such as Naive Bayes, Support Vector Machines, and Random Forests to learn patterns in training data and make predictions about the sentiment of new texts. This method is more flexible and adaptive than lexicon-based methods, and it can handle a wider range of sentiment expressions. However, it requires a large and diverse annotated dataset for training, and it may not be able to generalize well to new domains and languages.
Deep learning-based sentiment analysis
The third method is deep learning-based sentiment analysis, which involves using deep neural networks to model the complex relationships between words and sentiments in large-scale text data. This method has been shown to outperform other methods in many applications, and it can capture the rich semantic and syntactic features of text. However, it requires a massive amount of training data, computational resources, and expertise to develop and deploy, and it may not be suitable for resource-constrained or real-time applications.
In conclusion, sentiment analysis is a powerful tool for businesses and researchers to gain insight into the attitudes and emotions of people in online communities. The choice of sentiment analysis method depends on the specific requirements and resources of each application, and the best method may vary depending on the language, domain, and quality of the data. However, all three methods can provide valuable insights and opportunities for businesses and researchers to better understand and engage with their customers and audiences.